A young man fascinated by simple principles, and a woman drawn to the human side of research
Tanaka: I had computers at home from my childhood, and my love of computers led me to study computer engineering in university. After entering university, though, I spent most of my time playing football [soccer] and never thought I would become a researcher. When a professor from a corporate background started up a new research office doing simulations of communication and electronic devices, I decided to join that lab. Throughout the launch period of the lab, I experienced the fun of research and discovery.
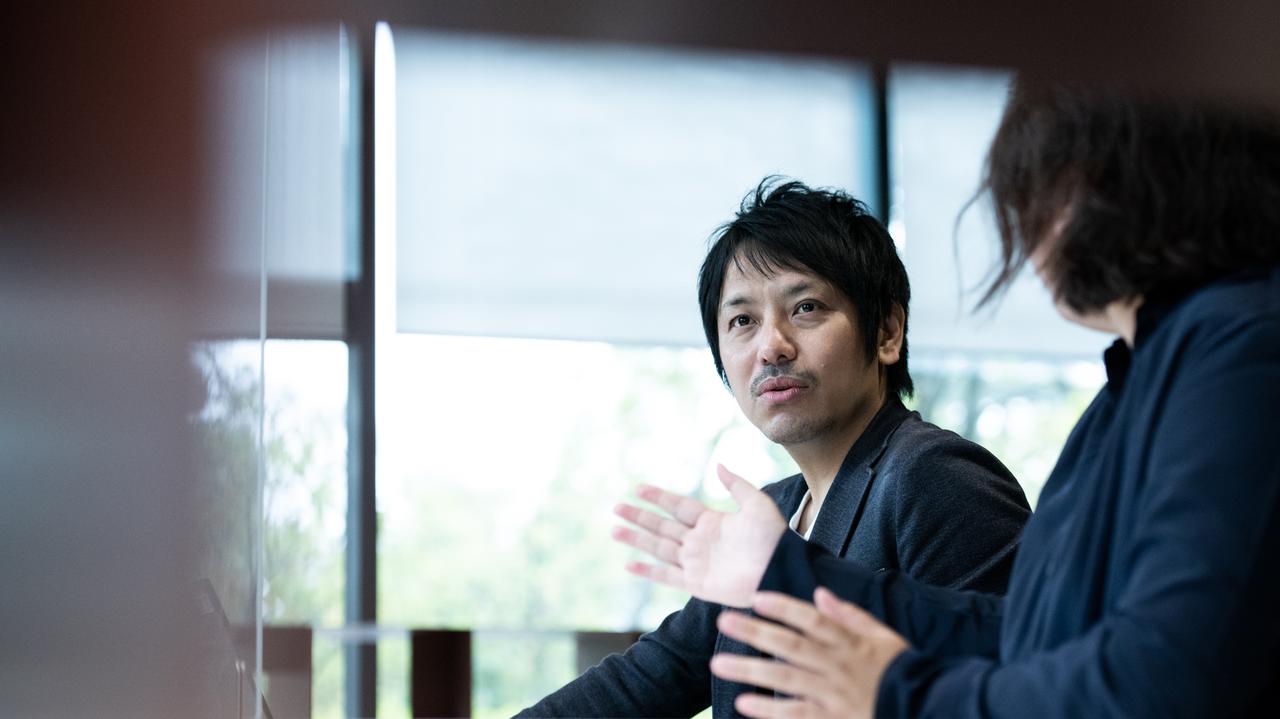
While my university life was devoted to playing football, I liked physics. Many physical phenomena can be expressed by simple principles. I felt that the same was true for simulations. It was fascinating to be able to express complex phenomena by expanding the scale of simple logic, and to be able to discover new phenomena using programs I wrote. Going on to graduate school, at the time job-hunting began I did not have a particularly strong sense of taking up a research career; but when I visited Hitachi, and saw the researchers working in such a free and relaxed environment, it looked interesting to me. Also, learning that Hitachi labs were carrying out research on sensor networks, at the stage prior to today’s IoT, I felt I would be able to put my knowledge of communication and electronic devices to good use, so I decided to join the company.
Ito: I dreamed of becoming an astronaut as a child, but gradually became more realistic as I grew up [laughs], thinking it would be nice to enter a profession in the sciences, such as a researcher or engineer. When I went to university, I decided to study medical system engineering in the Faculty of Engineering. The field of study I chose was biomedical engineering, conducting research in areas like medical devices, prosthetics, and power assist suits supporting surgeons. I think what attracted my interest was the idea of approaching medical care research from an engineering standpoint. Being from Akita Prefecture, I had planned on entering a university in the Tohoku region; but one thing that led me to go elsewhere was that my mother, a huge fan of the Chiba Lotte Marines baseball team, urged me to look at schools in the Kanto region.
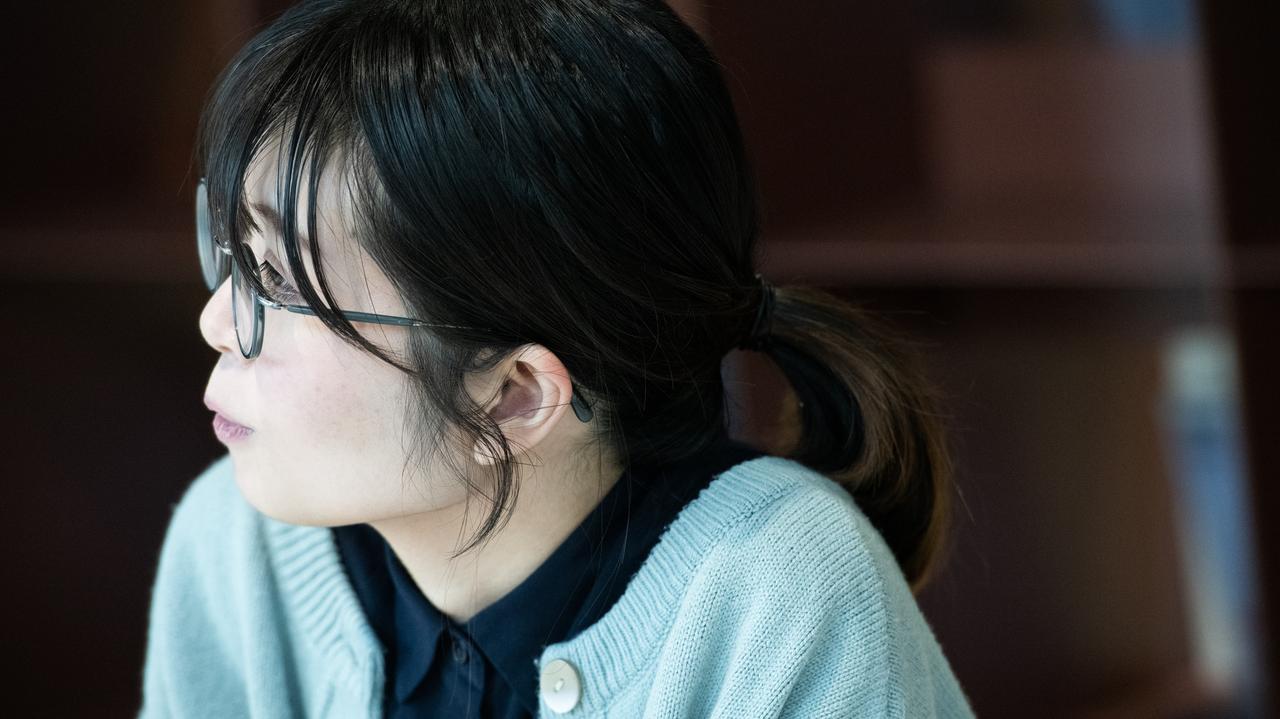
As a third-year undergraduate, I joined a laboratory led by a professor who was in the engineering department but was also a surgeon. There I engaged in research on using image processing technology to support cancer diagnosis with a colonoscope. This research, in a laboratory placing importance on the needs of doctors, was about using artificial intelligence (AI) to help determine whether cancer should be removed surgically. In graduate school, however, after I completed my first year in the master’s program, the lab I belonged to was discontinued because the professor I had been studying under moved on to another position. I spent my final year of the program in another lab that also was involved in image processing. There I was taught how to approach research from the standpoint of “seeds,” adapting technologies for application to real-life situations.
The result was that I got to experience two laboratories with opposite viewpoints, one starting from needs and the other from seeds. I learned that the value demanded from technology, and the way of thinking, are different in R&D and in the application field. I feel that I am drawing on that experience also in my current work.
In my job-hunting, pursuing a research career at Hitachi was my first choice. The main reason was that I thought many of the people doing research at Hitachi were interesting as people. I wanted to meet outstanding people and interesting people, and enjoy my research.
At present (fiscal 2022), I am in my fourth year at Hitachi. During my first year, taking up a research theme close to the medical image diagnosis and image recognition themes of my student days, I worked on developing an ultrasonic diagnostic system for breast cancer. In my second year, however, as part of business restructuring, the image diagnosis business was transferred to another company. For that reason, I became involved in research in a different field from previously. Having experienced the loss of my laboratory in university, this time I had a similar experience. That, however, was the occasion for coming to participate in my current research theme, the use of wearable devices and biosensors to predict truck driver fatigue.
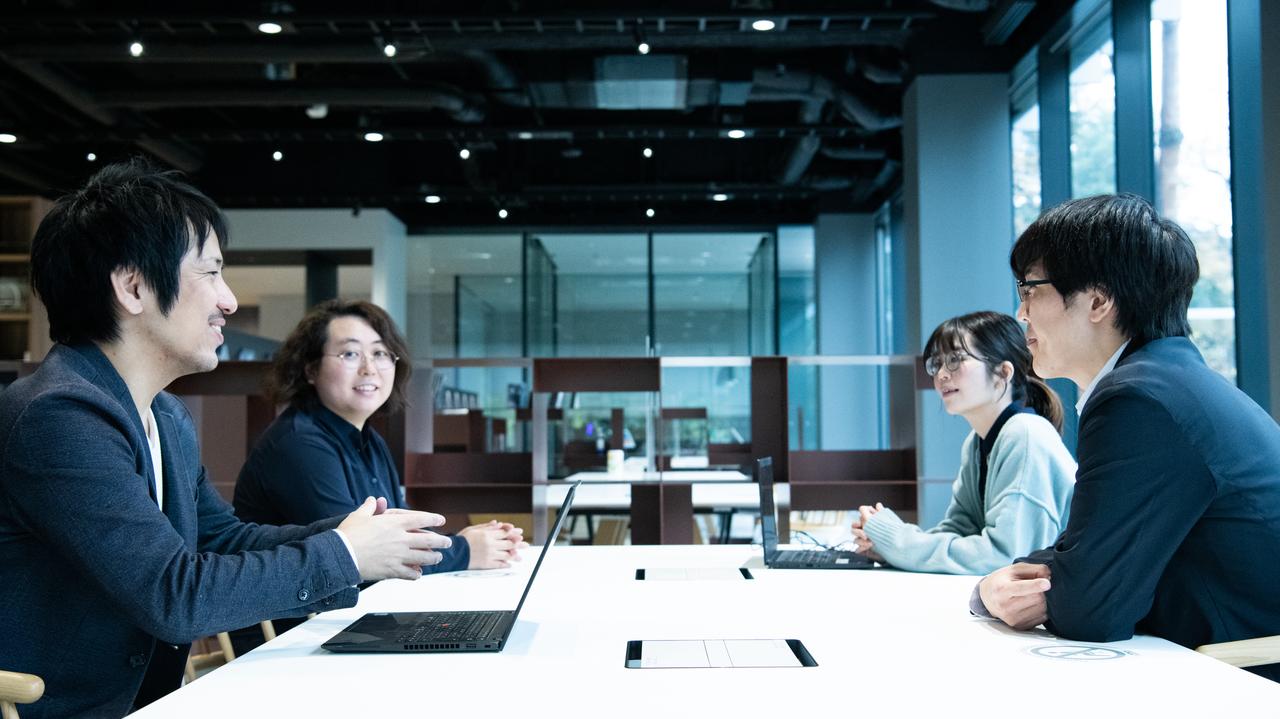
The beginning of research on applying biosensors to driver safety
Tanaka: After joining Hitachi, I got involved in research on gathering real-time information from people and things by connecting arrays of tiny sensors to a network, and experimenting with wearable devices that we made to measure heart rate and behavior. At that time, there was nothing like the watches and bands in use today to measure heart rate and behavior 24 hours a day, so this research was started up; and I take pride in being among the pioneers in developing quite early on something similar to today’s smart bands. The research itself was interesting, but unfortunately did not lead to a business in Hitachi.
For that reason, we began looking for a new research direction. That was research making use of sensor technology. We carried out many different studies on sensor use, such as for health promotion and lifestyle disease prevention, and for making efficient use of data on athletes’ movement in sports training. As for the scope of the sensor application, it tended in the healthcare direction.
In the course of these studies, we came to be involved in research on safety, aimed at preventing accidents by professional vehicle drivers. The impetus for this research was that, even though drivers operating vehicles daily at a Hitachi Group company underwent safety education and other measures were taken, accidents continued to occur. The idea was that by measuring driver fatigue and stress with sensors, it might be possible to prevent accidents in advance.
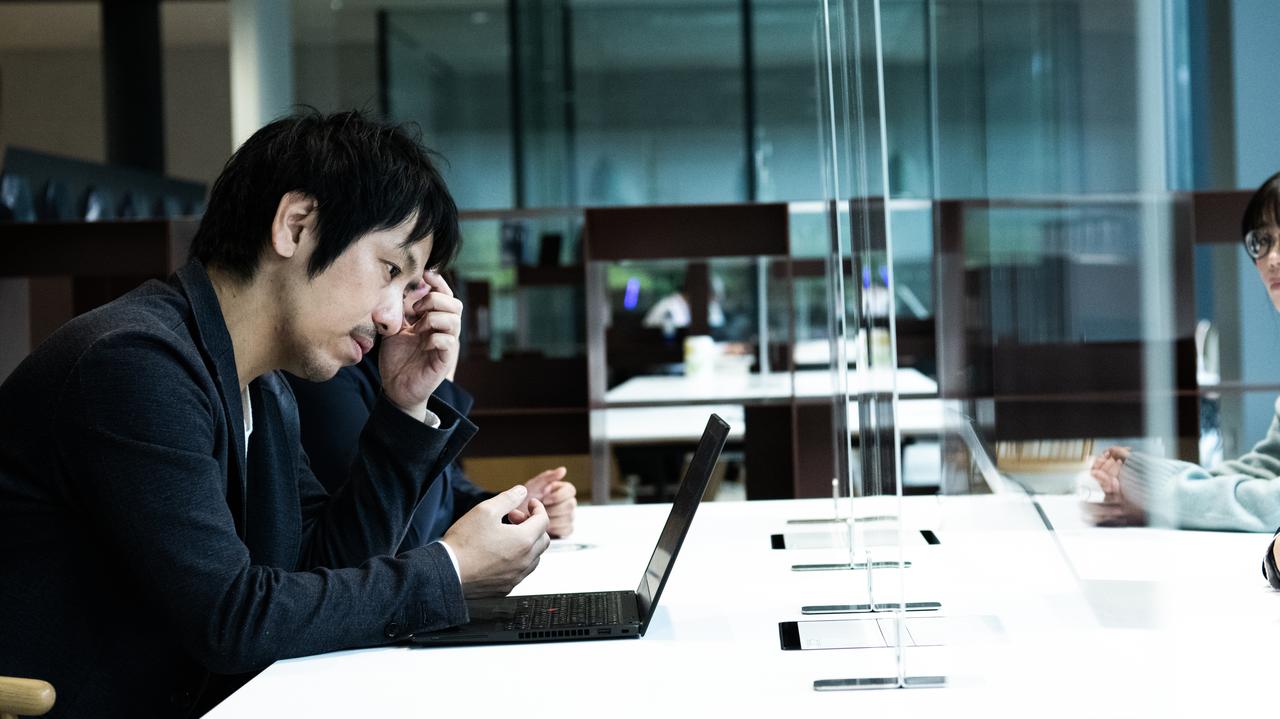
There had been an increase in accidents due to “inattentive driving,” whereby collisions resulted from lack of driver alertness, without a clear cause such as the driver being distracted or dozing off. A characteristic of such accidents is that, due to the lack of an obvious cause, it has been extremely difficult to devise countermeasures.
In studying the use of sensors for health maintenance and promotion, we worked on fatigue in close cooperation with prominent experts in that field, including Japanese Society of Fatigue Science board member Hirohiko Kuratsune, who has taught at Osaka University and Osaka City University, and President of the same academic society Yasuyoshi Watanabe, who is with RIKEN. At the time, there had been preliminary research on fatigue, but no scientific verification of its relationship to accidents or near miss incidents leading to accidents. Thinking that driver fatigue and changes in physical condition may be factors behind inattentive driving, we undertook the studies while receiving advice from these leading experts on fatigue research.
Then around 2017, we began prototype development. Starting in 2019, we asked Group company Hitachi Transport System (present LOGISTEED) for driving data for its 1,200 commercial vehicles, and for physical condition measurements by sensor data from a few dozen drivers. Based on the received data, we constructed a model of the relationship between inattentive driving and fatigue. We succeeded in elucidating the correlation between near miss incidents and fatigue as determined by physical condition measurements, and in devising an algorithm for this relationship. The results were written up in academic papers and published, enabling risk prediction based on scientific evidence.
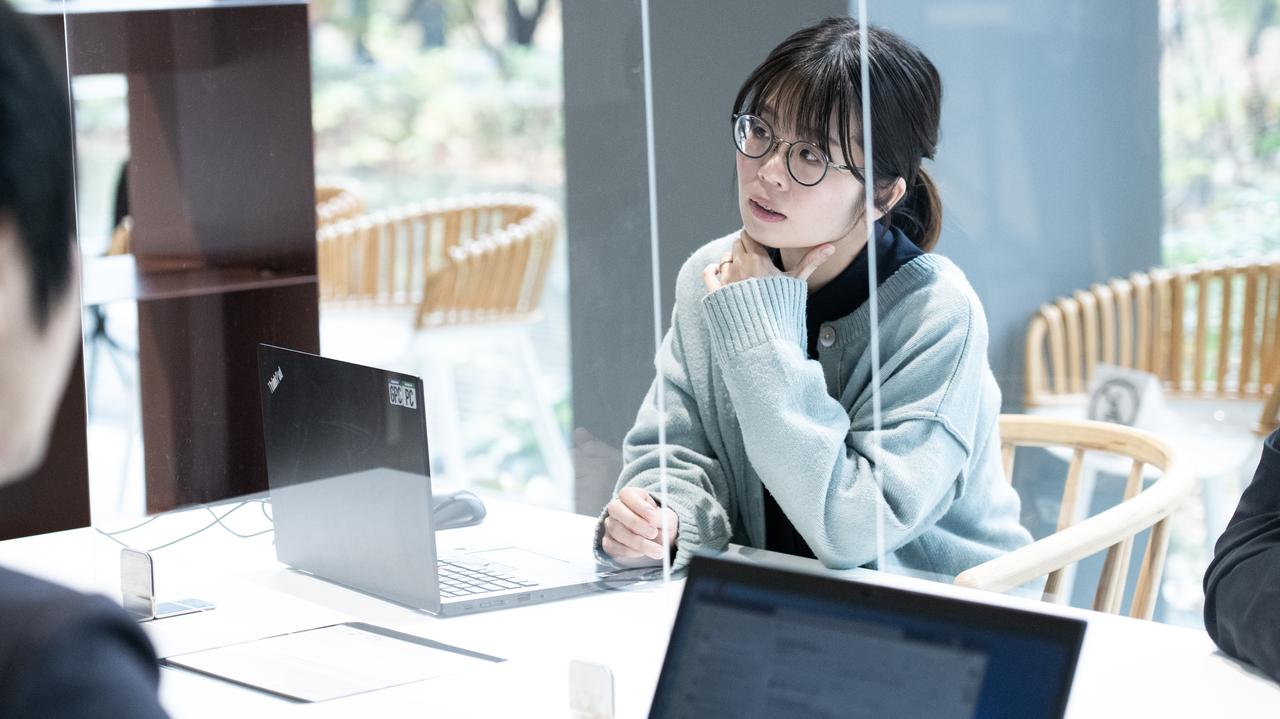
Ito: My own role, related to the part of the study using machine learning, was conducting research on the risk of accident occurrence using indicators to show fatigue, in which we tried to link physiological data to whether or not an accident occurred. We had driver’s physiological data measuring health conditions. We collected some 4,000 person-days of data from physical condition measurements before and after driving and clarified the correlation to accident risk.
With the aim of reducing accident risk from changes in physical condition, we collected 1,200 person-days of real-time physiological data and autonomic nerve measurement data during driving and elucidated the relationships between physical condition while driving and accident risk. By taking heart rate data, we were able to express various features indicating autonomic nerve function using heart rate variability analysis. When fatigue or stress occurs, even without the person realizing it, heart rate intervals change, linked to autonomic nerve activity. At the same time, we collected data also for vehicle behavior. Comparing changes in tens of autonomic nerve features and vehicle behavior, we investigated the correlation to near miss incidents.
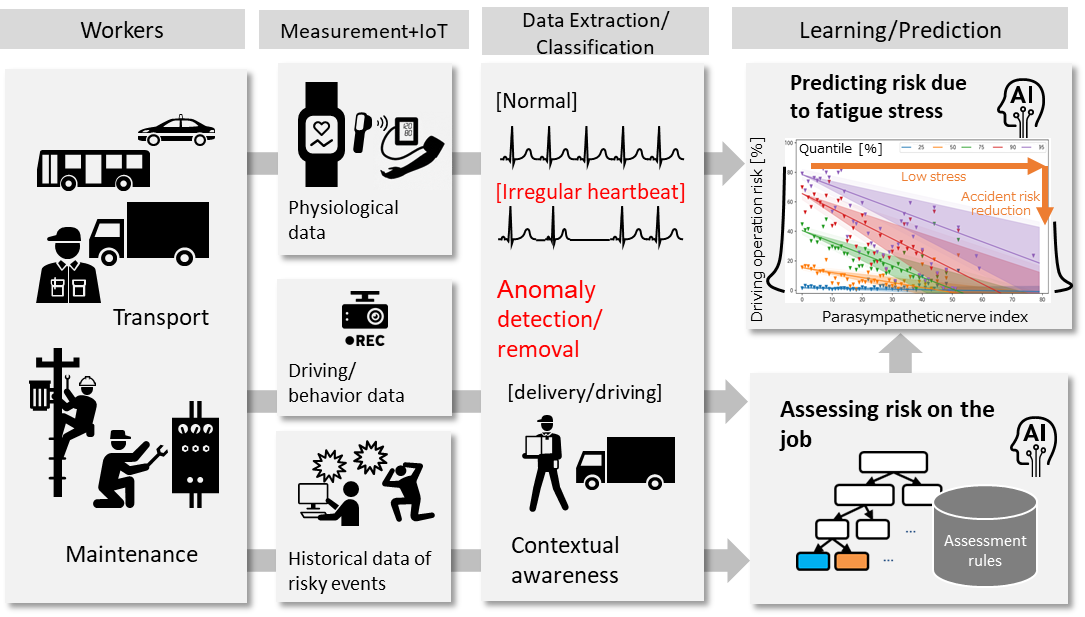
The reason we focused not on direct accident data but on correlation to near miss incidents is that in reality, accidents only rarely happen, making it difficult to obtain sufficient data. Instead of accident data, we investigated the correlation to near miss incidents, when scary situations are encountered, and developed an accident risk prediction algorithm.
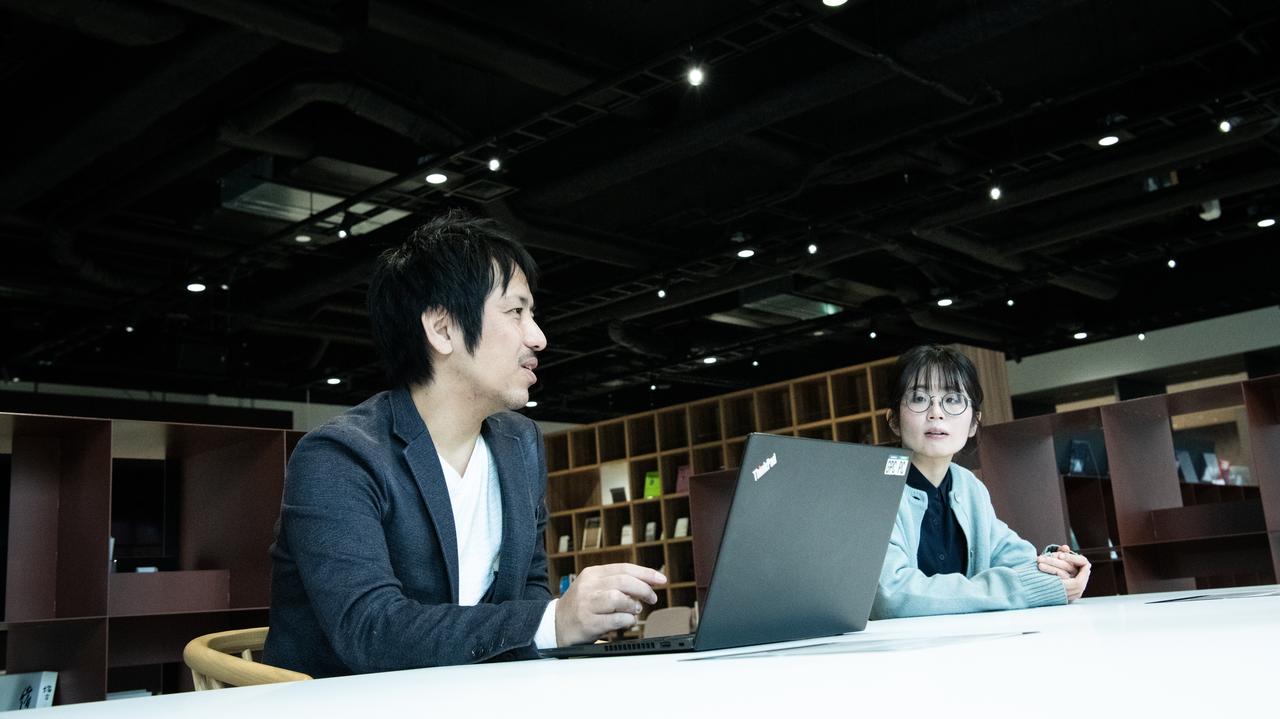
Tanaka: The results of our research were implemented in the operation safety management solution SSCV-Safety of Hitachi Transport System, which was also the impetus for our study. This is a safety assistance service that is part of the SSCV (Smart & Safety Connected Vehicle) solution for transport businesses. Besides being used in Hitachi Transport System itself, it is provided outside the company as a solution, and is used in logistics safety initiatives aimed at zero accidents.
SSCV-Safety uses sensors to measure heart rate and other biometric information and predict the likelihood of fatigue or accident occurrence. The functions correspond broadly to the three stages “pre-shift,” “during shift,” and “looking back.” At the “pre-shift” stage before entering the vehicle, the driver’s physiological data is measured to predict the occurrence of near miss during driving due to physical condition or fatigue, leading to a reduction in accident risk. In the “during shift” stage while driving, physiological data is obtained in real time, notifying the driver and management of dangerous situations. In the “looking back” stage, near miss incidents detected by AI or devices are confirmed by video images, and the situation is fed back at the end of the workday. I am truly happy that as a result of our having continued research with Hitachi Transport System, the provision of SSCV-Safety has begun.
Tanaka: The theory that driver fatigue was a cause of inattentive driving has been around for some time, especially among those working in the logistics field. What was lacking, however, was knowledge about how to measure fatigue and feed it back. In our research, we developed technology for classifying near misses by machine learning, and technology for real-time prediction of fatigue-caused accident risk from physiological data.
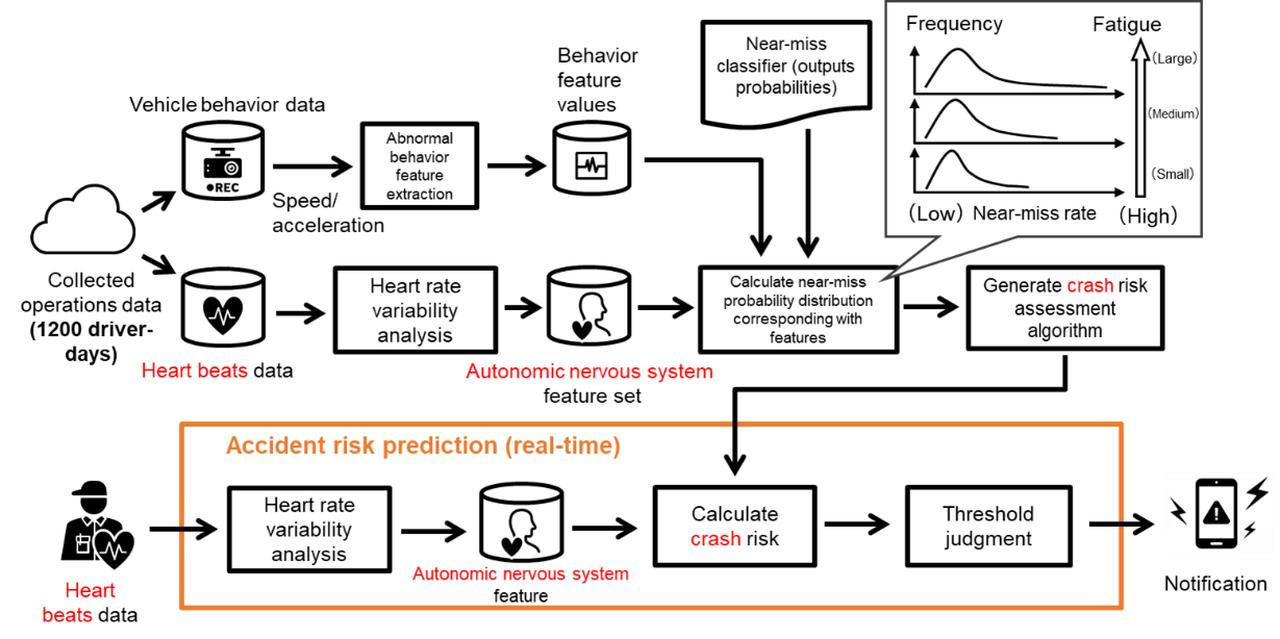
We first extracted the characteristics of abnormal behavior from vehicle behavior data, then developed near miss classification technology for outputting the risk level at which near miss incidents are relatively likely to occur. It is possible to confirm near miss incidents by human sight from in-vehicle camera images, but this is highly time-consuming. We therefore used machine learning so that near miss incidents could be classified automatically.
Next, to enable real-time prediction of accident risk due to fatigue from physiological data, we analyzed the relation between heart rate data for 1,200 in-service driver person-days and near miss incidents. From this analysis, we sought to derive approximately 50 features of autonomic nerve functions. It turned out to be difficult to determine correlations directly from features such as fatigue and stress obtained from heart rate data and the number of near miss incidents. We therefore used risk distribution to calculate the relation between features and near miss incident likelihood (risk level). From these results, we discovered the tendency for the probability distribution in states of particularly high risk level to spread, depending on specific fatigue, stress, and other features.
In other words, if features pushing the distribution of near miss incident risk level toward the low end can be extracted, we should be able to find out driver states in which accident risk is low. In this research, we found that by controlling the extracted parasympathetic nerve activity index (HF) in the low-stress direction, the probability of being in a high risk level state can be reduced by up to 50 percent.
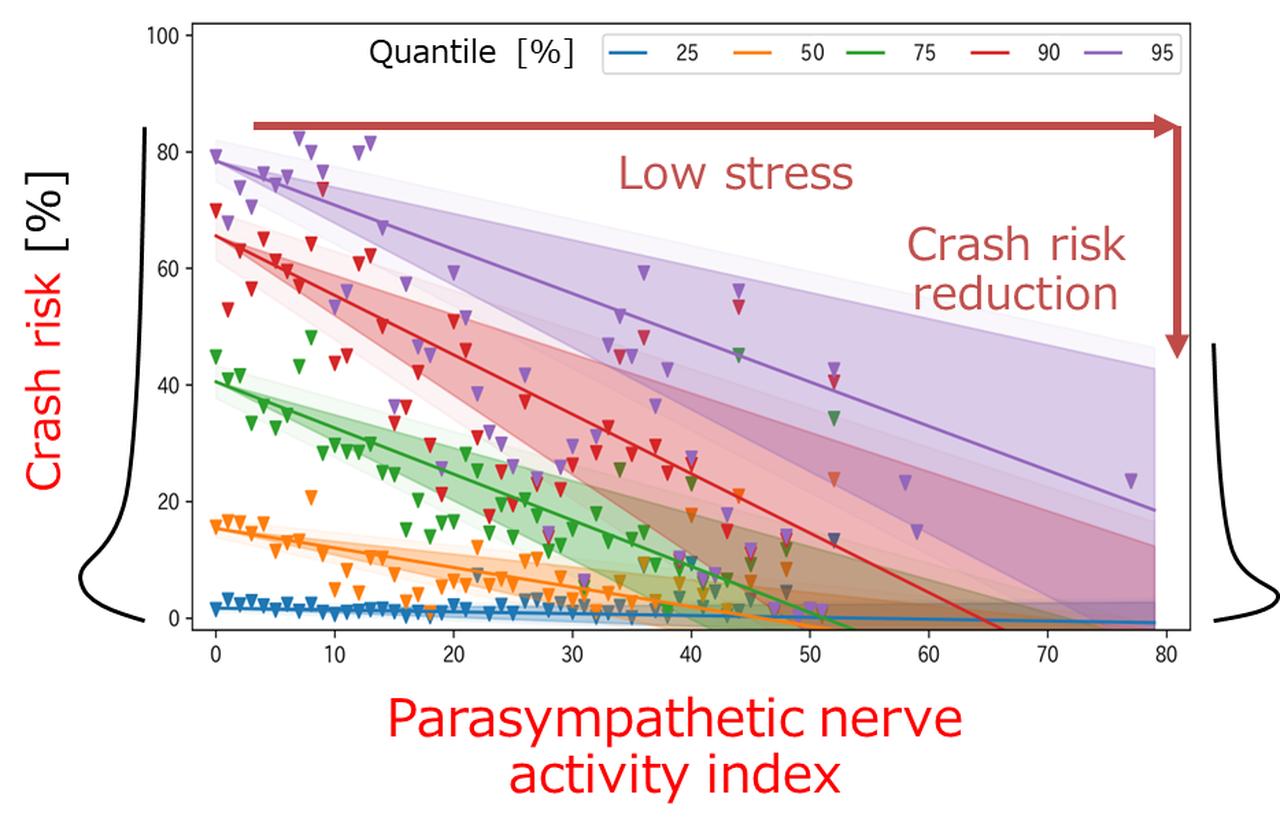
Ito: We had a tough time measuring physiological data in real time. The best way of measuring heart rate data is with the subject at rest with eyes closed. Real-time data while driving, however, cannot be taken in such a state. We tried developing a method using a wristband-type device applicable while driving, but we ran into endless difficulties, as data could not be obtained reliably due to vehicle vibration or steering wheel operations and the like, and data was delayed or missing when radio reception was bad.
Tanaka: The research itself is new. Besides there being a lot of noise in physiological data, and large variations across individuals, the frequency of near miss incidents is not all that high. The correlation cannot be expressed simply. I believe we were successful in modeling the correlation of physiological data features to the probability distribution of near miss incidents by approaching the problem both from that of discovery while viewing data, and from how to model that data.
Ito: With the technology used in developing SSCV-Safety, we learned that high-risk situations are reduced when the values for parasympathetic nerve activity index (HF), which is said to be dominant among autonomic nerve activities when in a relaxed state, become larger. This is a simple index, and was endorsed by the fatigue experts who advised us in this project. In this way, we discovered the autonomic nerve features for which accidents trend lower. Actually, though, it would be better still if a warning could be issued when a high risk level was detected. It proved difficult, however, to accurately discover with just one indicator the circumstances in which risk level rises.
We therefore studied the use of deep learning technology, able to grasp complex events without simplifying, for discovering relationships between multivariable features and risks. By using a model that combines complex indicators and performs nonlinear feature propagation, we became able to predict accident risk with around 80 percent accuracy. Deep learning tends to be a black box, though, so we are continuing with research toward making the model decision criteria and indicators visible, enabling drivers to better understand why an alert of high risk was issued.
Meanwhile, we are currently working on making the measurements a bit simpler, and trying to figure out ways of refining the product so it can be offered to many different companies. This has been the direction of our research for the past year, as we study preprocessing of noisy data and working on evaluation methods, so that we can achieve simple and convenient measurements during driving. In the Hitachi Transport System case, we applied technology from R&D for protecting driver safety; but we are continuing with research aimed at expanding the technology for estimating accident risk from physiological data to other fields of work, such as dangerous equipment maintenance.
Tanaka: Adoption of the technology we developed in Hitachi Transport System’s SSCV-Safety is one result, but in the future we hope to expand it to other industries where human work that entails danger is necessary, so that it can solve many problems in society.
Connections between interesting people as equals supports research
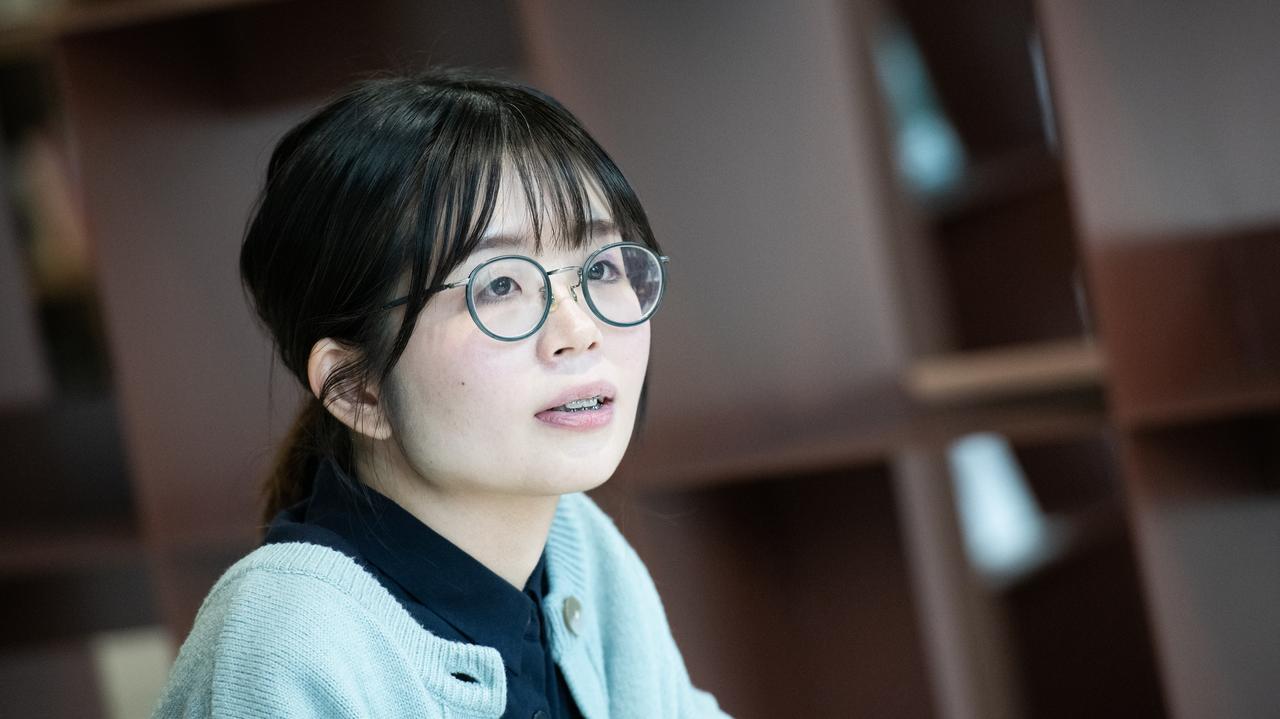
Ito: Doing research at Hitachi, I feel the wealth of human talent. I met people at a number of companies during my job hunting, but at Hitachi I was impressed with how people engaged with each other as equals. In talking with students visiting for job interviews, as well, they treated us earnestly. This attitude has not changed even after I joined the company, as in a team with a wide range of ages and skills, even the views of young people are listened to respectfully. Come to think of it, before I started working, I somehow imagined that when I joined a company I would bump against the seniority system or even encounter harassment. In fact, though, it seems to be a feature of Hitachi that a generation gap in the bad sense is lacking, as people tend to show mutual respect across age barriers.
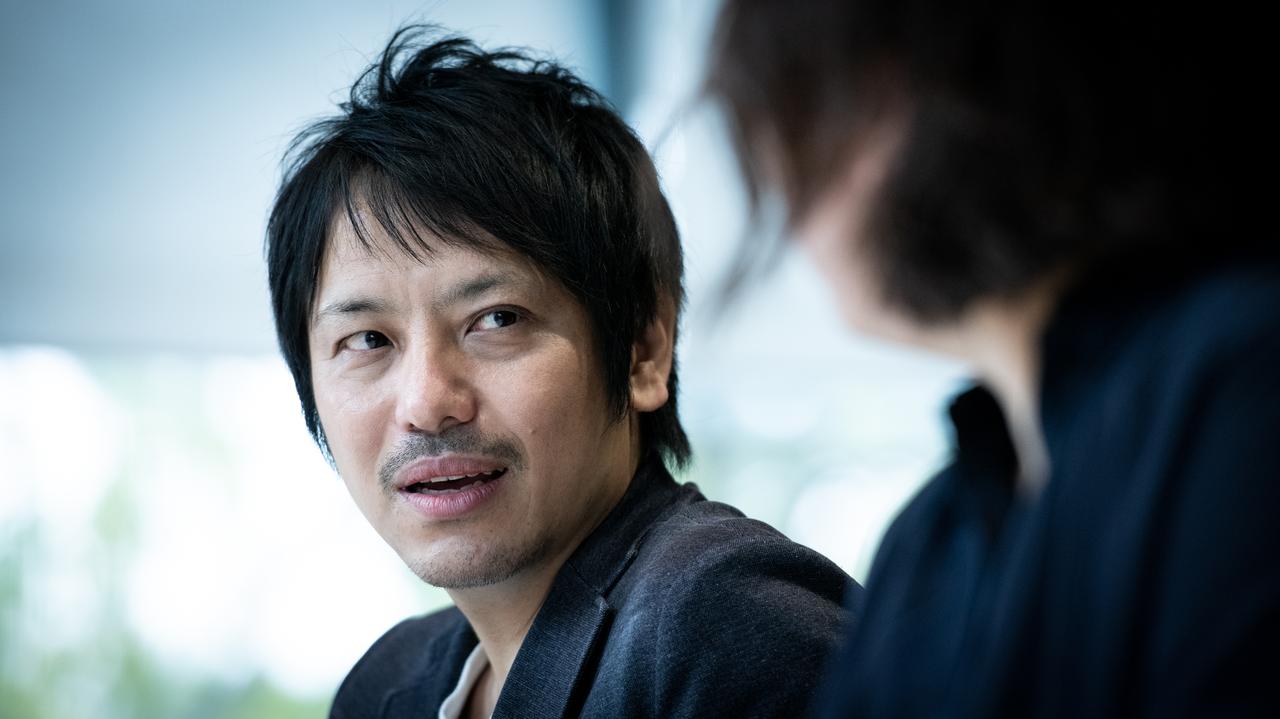
Tanaka: In Hitachi, we work with a wide range of people, including researchers and designers, in the various business divisions. The broader the spread, the greater breadth there is to ways of solving problems. What’s more, as research is conducted across a wide range of topics, the number of business areas grows, such as health, public sector, and medical care, and various connections between them become possible. Even if nothing results from these connections immediately, there are cases where something clicks after coming full circle over a period of years, and solutions to research problems are found.
It is definitely true that people at Hitachi tend to be unpretentious and open to others regardless of age, position and so on. I find many of them to be kind and easy to talk with, which may help explain why the environment is so conducive to personal connections.
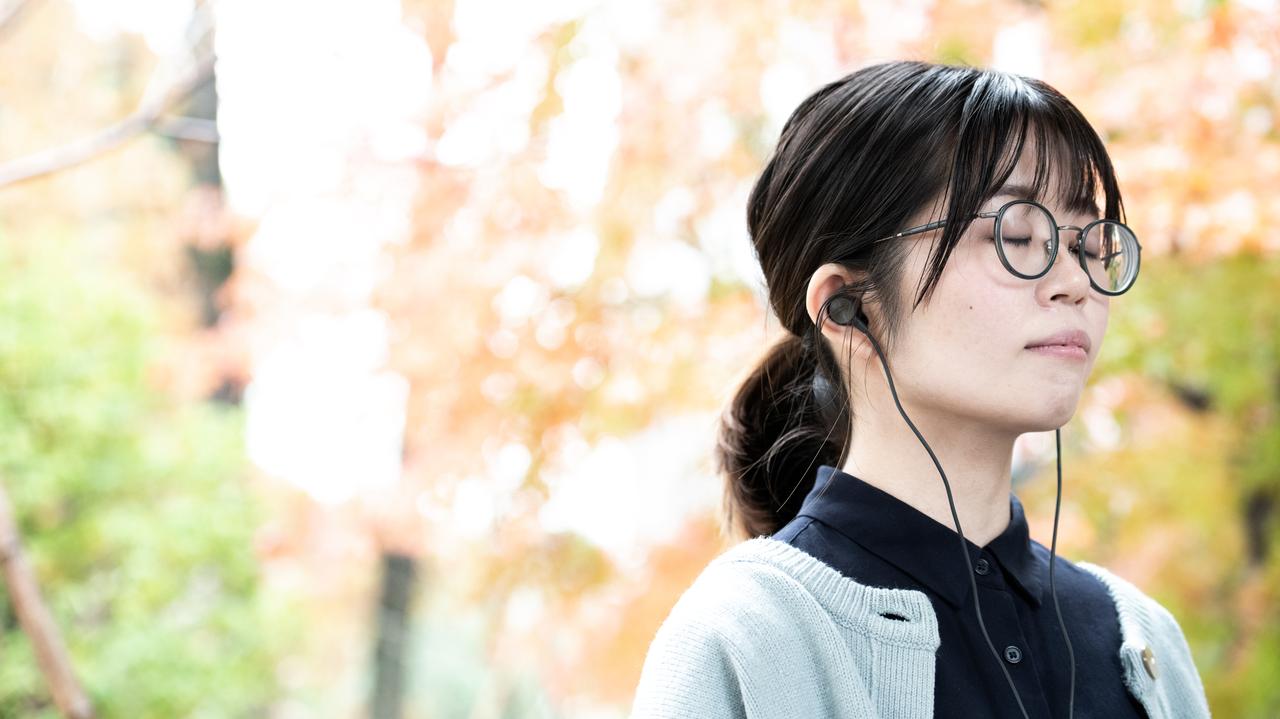
Nao ITO
Reseacher
Digital Healthcare Research Department
Healthcare Innovation Center
Center for Digital Services
RESEARCH & DEVELOPMENT GROUP
A song that inspires me
I love the music of Shiina Ringo. One song of hers I listen to a lot is “Life is full of dreams.” It’s a song that stuck with me while I was struggling to write my master’s dissertation. The lyrics, first of all, are really nice. The song resonates with me, now that I am an adult, when I am agonizing about how to make or represent something. Often these days, people can’t even tell whether or not you have put a lot of time and effort into something; but the idea is to carry on with your work earnestly for the sake of those who can tell the difference. The song has an unusual composition, and I would like people to give it a listen. It’s music I encountered as a student, but now that I am in the working world, this song shines a light when, during my work, I lose sight of where I am and what I am supposed to be doing.
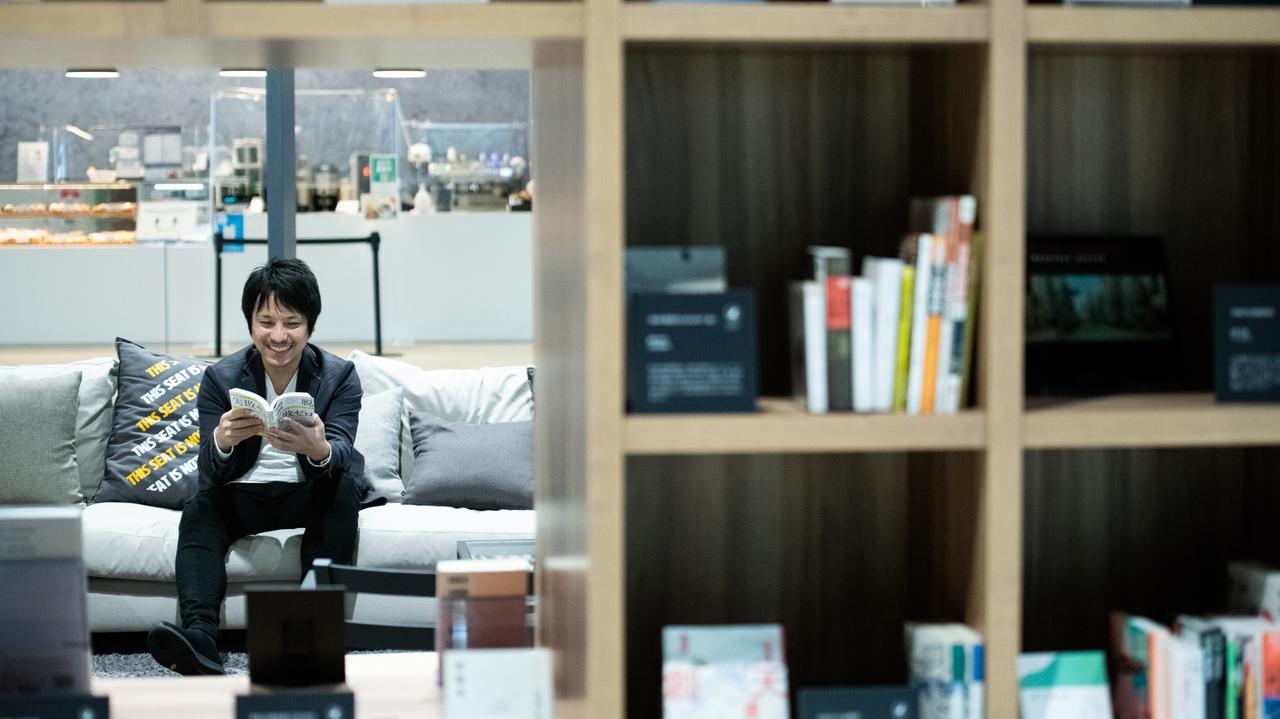
Takeshi TANAKA
Chief Researcher
Digital Healthcare Research Department
Healthcare Innovation Center
Center for Digital Services
RESEARCH & DEVELOPMENT GROUP
Learning from the works of Kenji Miyazawa how to capture the natural world
I found the Complete Works of Kenji Miyazawa (Chikuma Shobo) important to me among Japanese literature. The pocket edition of the complete works is 10 volumes. I read all of them when I was in graduate school, and still have them. Kenji Miyazawa was also a science teacher, who put into his writings a scientific way of capturing the natural world. I feel that in depicting natural phenomena in an interesting and ironic way, the interpretations of nature that he expresses based on an understanding of it are close to a research model. Like many others, I am a fan of his “Night on the Galactic Railroad” and “Spring and the Demon.” I am impressed by the way he captures natural phenomena just as they are, accepting them in the midst of pain and expressing them.
I would also like to introduce one more work, “Breaking free from zero failure” by Shigeru Haga (Kadokawa). This book is about an area somewhat related to my present work. As is now known, the former approach to safety of “zero accidents” led to extreme measures that made the work environment a difficult place to work and had other harmful effects. The “resilience engineering” explained in this book is a new approach to safety that is drawing attention. The aim of this approach is to “maximize the things that can be handled flexibly on a regular basis.” Since this provides us researchers with a new viewpoint, it is a highly useful reference.