Aimed at enhancing natural language reasoning capabilities in generative AI to support advanced decision-making
Hitachi has developed core technology for automatically generating training data (logical thinking datasets) to enhance the logical thinking capabilities of generative AI. This technology opens new possibilities for addressing complex decision-making questions, such as "Should our company invest in the XX business in the YY region?" by leveraging natural language reasoning capabilities. Hitachi will continue to evolve this technology through customer collaboration, aiming to develop generative AI that supports solving complex societal challenges.
Generative AI excels at utilizing existing knowledge to support routine tasks. Recently it has even become capable of solving more advanced problems such as mathematics and programming. However, it still lacks sophisticated logical thinking capabilities in natural language, failing to handle high-level decision-making such as market entry strategies and leading-edge technology investments.
Building on established expertise,*1 Hitachi has developed core technology that automatically generates logical thinking datasets. The technology produces high-quality data incorporating multi-step reasoning processes required for complex problem-solving and diverse thinking patterns based on mathematical logic. Moreover, by adopting an open approach, this technology can enhance any generative AI system through additional training (Figure 1).
Hitachi verified this technology with the most advanced generative AI system,*2 showing improvement in logical reasoning capabilities by an average of about 9% and up to 30%. Mathematical and programming capabilities also showed significant enhancement (Figure 2).
Hitachi will present this technology at the Thirty-Eighth Annual Conference on Neural Information Processing Systems (NeurIPS 2024), the premier international academic conference in artificial intelligence, on December 10-15, 2024, in Vancouver, Canada.
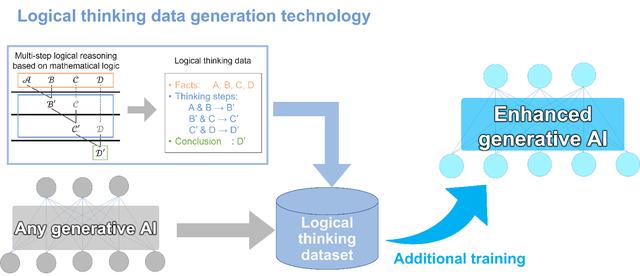
Figure 1. Enhancement of logical reasoning capabilities in generative AI through additional training with logical thinking datasets
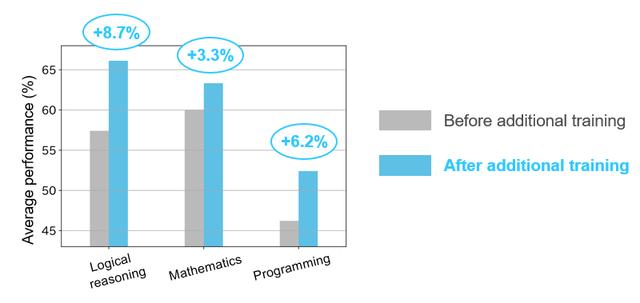
Figure 2. Performance improvements in generative AI*2 from additional training on logical thinking datasets
*1 List of papers and presentations to date
- Terufumi Morishita, Gaku Morio, Atsuki Yamaguchi, Yasuhiro Sogawa, "Learning Deductive Reasoning from Synthetic Corpus based on Formal Logic" Proceedings of the 40th International Conference on Machine Learning, 2023/07.
- Terufumi Morishita, Atsuki Yamaguchi, Gaku Morio, Hikaru Tomonari, Osamu Imaichi, Yasuhiro Sogawa, "JFLD: A Japanese Benchmark for Deductive Reasoning Based on Formal Logic" Proceedings of the 2024 Joint International Conference on Computational Linguistics, Language Resources and Evaluation, 2024/05.
*2 As of November 2024, based on Hitachi survey. As one example, LLaMa-3.1-70B was used.
For more information, use the enquiry form below to contact the Research & Development Group, Hitachi, Ltd. Please make sure to include the title of the article.
https://www8.hitachi.co.jp/inquiry/hitachi-ltd/hqrd/news/en/form.jsp