Contributing to the realization of sustainable industries by constructing manufacturing processes for low-environmental-load material utilizing organisms
Hitachi has developed a technique for identifying the optimal metabolic pathway to produce useful substances by virtually creating tens of thousands of series of enzymatic reactions (metabolic pathways) through artificial intelligence (AI). We have introduced the mechanism of biological evolution to an AI that has learned the biochemical reactions of various substances. Conventionally, metabolic pathways have been identified by manually repeating biochemical experiments since there are no specific rules for change in material structure under chemical reactions, and the developed technique makes it possible to efficiently explore and design metabolic pathways on a computer. By continuing to combine this information technology (IT) with control and operation technologies (OT) such as plant control technologies, Hitachi will contribute to the realization of sustainable industries, such as the construction of manufacturing processes for low-environmental-load material utilizing organisms.
Background and issues addressed
- Recently, attempts to realize sustainable industries by creating biological cells that are artificially optimized for material production and applying them to the production of substances have attracted much attention.*1
- To create such optimal cells, metabolic pathway design, which modifies a series of biochemical reactions (enzymatic reactions) occurring in a cell to produce new substances or increases the amount of production, is necessary.
- Because there are more than ten thousand possible combinations for enzymatic reactions that occur in cells, identifying the metabolic pathway to produce the target substance has conventionally required substantial trial and error in manual biosynthetic experiments by biochemistry specialists.
- To reduce time and cost in creating cells through synthetic experiments, an efficient technique for exploring metabolic pathways utilizing digital technology is strongly sought.
Developed technologies
- AI to predict structural changes of chemicals before and after enzymatic reactions
- Technique for exploring metabolic pathways to optimize combinations of enzymatic reactions by imitating mechanisms of biological evolution
Confirmed results
- The effect of the developed technique was verified in the identification of biosynthetic reactions that are not listed in the database used in AI training, and it was confirmed that one of the 2-butanone*2 biosynthetic reactions predicted by this technique was consistent with the biosynthetic reaction reported in the journal.*3
- This result indicates the possibility of predicting the existence of biochemical reactions that are not included in the data, that is, feasible metabolic pathways that can be realized by an organism.
Published papers, conferences, events, etc.
This achievement was presented at the 19th European Conference on Computational Biology (ECCB2020) held online from September 7 to 8, 2020, and will be published in the scientific journal "Bioinformatics" (publisher: Oxford University Press). (Title: Feasible-Metabolic-Pathway-Exploration Technique using Chemical Latent Space).
Acknowledgements
This paper is based on results obtained from a project named “Development of Production Techniques for Highly Functional Biomaterials Using Plant and Other Organism Smart Cells” commissioned by the New Energy and Industrial Technology Development Organization (NEDO).
Details of developed technologies
1. AI to predict structural changes of chemicals before and after enzymatic reactions
Conventional methods for handling enzymatic reactions on a computer have used reaction rules manually prepared to determine structural changes such as increasing or decreasing number of molecules and partial structural changes. However, because it is difficult to cover all the molecular positions and substructures of a compound with diverse structures, it is not possible for conventional methods to correctly predict the structure of the compound after an enzymatic reaction has occurred. In this study, we first developed an AI to predict compound structural changes before and after an enzymatic reaction, focusing on the idea that enzymatic reactions can be expressed using a mathematical rule of vector arithmetic operation by using the deep generative model*4 of compound structures. By adding or subtracting a vector indicating an enzymatic reaction, it is possible to predict the structure of a compound after an enzymatic reaction, even if the reaction has not yet been discovered.
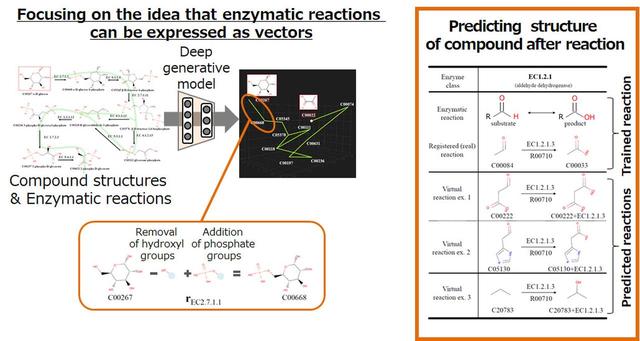
2. Technique for exploring metabolic pathways to optimize combinations of enzymatic reactions by imitating biological evolution mechanisms
The design of metabolic pathways must consider known enzymatic reactions that occur in cells as well as undiscovered enzymatic reactions that can potentially occur in cells. To identify feasible metabolic pathways in cells from a number of combinations of enzymatic reactions, we have developed a technique for exploring metabolic pathways that mimics the mechanisms of biological evolution. First, known enzymatic reactions are converted into vectors using the AI described above and stored as a database in the system (enzymatic-reaction feature DB). Next, combinations of enzymatic reactions are randomly selected from the database, and the validity of the reactions, such as whether the target substance can be produced by the selected combination of the reactions (metabolic pathway candidate) or whether it can occur in the cell, is evaluated. Low-valid combinations are discarded while only high-valid combinations are retained as candidates, and reactions are exchanged between the high-valid combinations. By repeating these processes, we have made it possible to identify a highly feasible metabolic pathway to produce a target substance in a cell.
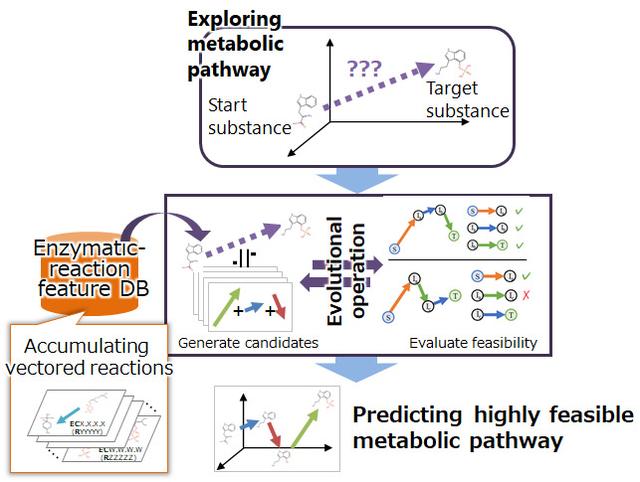
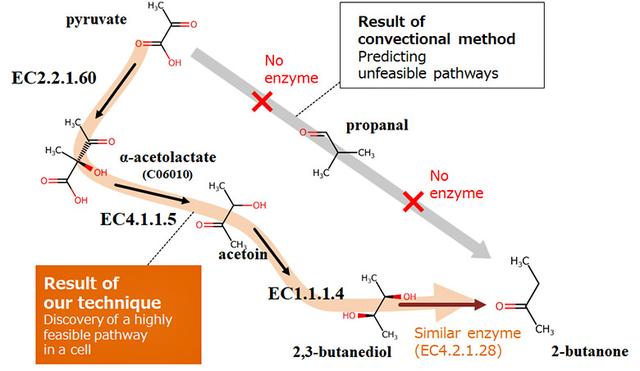
*1 "Carving Out a Future with Biotechnology and Digital Technology Smart Cell Industry, Efforts Towards Open Innovation", Focus NEDO No. 70.
*2 2-Butanone: a raw material for paint solvents and synthetic resins.
*3 Chen, Zhen, et al. "Metabolic engineering of Klebsiella pneumoniae for the production of 2-butanone from glucose." PLOS ONE 10.10 (2015): e0140508.
*4 Jin, W., et al., "Junction tree variational autoencoder for molecular graph generation" in International Conference on Machine Learning (2018)., pages 2323–2332.
For more information, use the enquiry form below to contact the Research & Development Group, Hitachi, Ltd. Please make sure to include the title of the article.
https://www8.hitachi.co.jp/inquiry/hitachi-ltd/hqrd/news/en/form.jsp