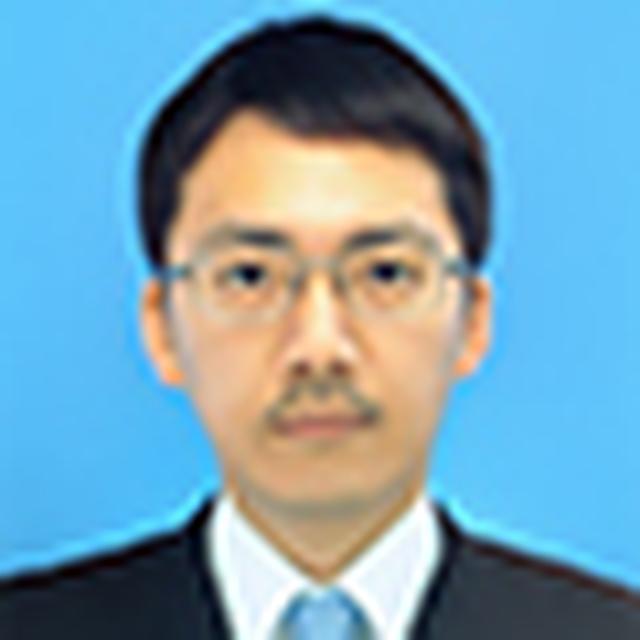
Takuya Kanazawa
Research & Development Group, Hitachi, Ltd.
Innovation in materials has the potential to transform the society. For example, recyclable plastics could lead to a waste-free society, and advanced superconducting materials could enable new communication and cryptography systems. Investment in materials science and engineering is rising, notably as part of national initiatives in several countries. The Materials Genome Initiative *1 of the United States is a particularly famous example. In recent years, materials informatics (MI) has gained popularity as a data-driven approach to materials discovery and design %%*2{sup}%%. While the success of MI hinges on materials datasets with wide coverage and high accuracy, such datasets are not yet sufficiently available in many fields of physics and chemistry. Thus, the demand for high-cost manual experiments to garner new data remains high. Precise determination of material properties often requires a sequence of elaborate measurements, and it is highly desired to expedite such experiments in order to reduce the time, money, and effort involved.
We explored optimization strategies for sequential experiments in materials science, taking the small-angle neutron scattering (SANS) experiment as a representative example. SANS is widely used to study the microstructures of various materials including alloys, ceramics, and polymers, but the design of an optimal SANS experiment has been left to manual adjustment by experienced researchers. To alleviate this burden, we formulated the design of a SANS experiment as a multi-step decision making problem and introduced two methods that enable adaptive measurement planning. The core idea is to create a database of virtual experiments by means of simulation and then use it adaptively during the real experiment to determine the target of the next measurement. In the first method, we use simulation to fix the best sampling procedure for each of many virtual samples and record the procedures in a database. During the actual experiment, we send the acquired data into a similarity search over the database and retrieve the best sampling protocol for the most similar sample. In the second method, the outcomes of future measurements during an experiment are predicted in two distinct ways: one uses the database of simulated measurements, while the other does not. The target of the next measurement is then determined through comparison of the two predictions.
To benchmark the methods, we prepared 100 virtual sample materials and simulated SANS experiments on them. The goal of the experiment is to estimate the particle size distribution inside the material from the neutron scattering intensity. The experimental duration required to achieve satisfactory accuracy was reduced by 50% – 65% with these methods, as compared to random sampling with no prior planning.
In Figure 1, we show the process of data sampling with our second method for one sample material. The accuracy of the estimate for the particle size distribution (right column) improves rapidly as the number of measured points increases. Since the small-angle X-ray scattering (SAXS) also shares the same principles with SANS, we believe our methods will work for SAXS experiments as well.
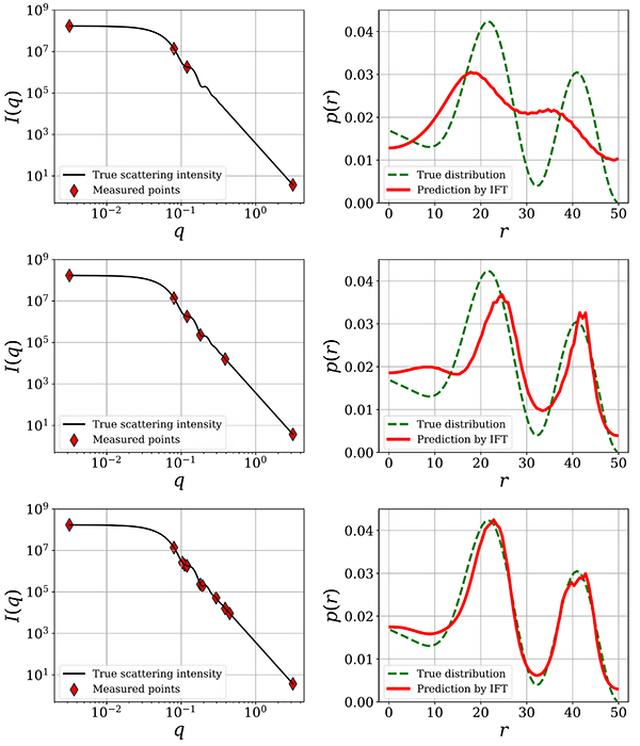
Figure 1: Example of a measurement process with our new method.The left column is the scattering intensity and
the right column is the particle size distribution (both the ground truth and the predicted one).
The number of sampled points is 4 (top row), 6 (middle row) and 12 (bottom row), respectively.
In summary, we have developed a novel methodology to accelerate high-accuracy measurements of material properties. This work will contribute towards high-throughput materials discovery and design. For further details of our work we refer to the original paper available at https://doi.org/10.1088/2515-7639/ab3c45.
Acknowledgements
Thanks to my co-authors Akinori Asahara and Hidekazu Morita with whom this research work was jointly executed.
References
*1 J. J. de Pablo et al., “New frontiers for the materials genome initiative,” NPJ Comput. Mater. 5:41, 2019.
*2 R. Ramprasad et al., “Machine learning in materials informatics: recent applications and prospects,” NPJ Comput. Mater. 3:54, 2017.