Increases processing speed by an order of magnitude, toward more efficient data storage in data centers and cloud services
Hitachi has developed a new lossless data compression*1 technology that uses deep learning to improve data storage efficiency. The technology, adopting an originally developed high-speed inference model that is not dependent on the number of deep learning layers, is not only capable of high-accuracy prediction of the data sequence patterns*2 necessary for data compression but significantly reduces processing time. As a result, while maintaining the same level of prediction accuracy as conventional inference model approaches, the inference model processing speed was confirmed to be approximately an order of magnitude faster.*3 This technology is expected to improve the data storage efficiency of data centers and cloud services while easing the economic and environmental burden of customers.
The increasing volume of digital data has made storage costs a major issue for customers, one that companies typically address by using data compression technology. Conventional lossless data compression is able to restore the data fully to its original form, but compression efficiency is limited by the inability to optimize the characteristics of different types of data, such as text and images. The hope was that compression technology using deep learning would be capable of optimization based on the characteristics learned from data. Because of the lengthy processing time of conventional deep learning technology, however, this approach was not suited to business applications.
The newly developed lossless data compression technology, drawing on Hitachi’s expertise in areas such as deep learning models and data storage, makes use of a high-speed inference model for which the processing time is not dependent on the number of deep learning layers. Specifically, the inference model, for which long computing time was an issue, is designed so that computing time does not increase with an increase in number of layers, by saving in each layer the intermediate results for that layer and calculating in one batch operation. In this way, it became possible to significantly reduce the inference model computing time (Figure 1).
A comparison of the new technology and the conventional inference model method under defined conditions shows that similar prediction accuracy is achieved at processing speeds approximately an order of magnitude faster than the conventional approach. This technology, making possible fast and efficient storage of vast amounts of data in data centers and cloud services, is expected to ease the economic and environmental burden of customers.
Hitachi will continue to carry out research and development towards even faster processing and seek to achieve practical implementation of the new technology through collaboration with customers using data centers and cloud services.
This technology was introduced in part in the November 2024 IEICE Transactions Online.
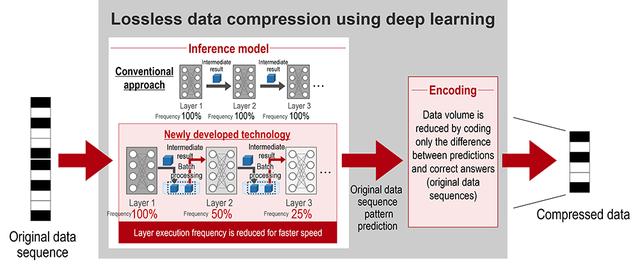
Figure 1. An overview of lossless data compression and the newly developed technology making inference model processing faster by an order of magnitude
((1) Data sequence patterns are predicted with high accuracy by an inference model using deep learning, (2) the intermediate results for each layer are saved in that layer and batch calculated, (3) reducing the frequency of calculation at deep layers, so that processing volume does not increase even with an increase in layers.)
*1 Lossless data compression: Data compression technology that compresses the size of data without any loss of the original information. Since the compressed data can be restored to its original state, this technology is used when data consistency is important.
*2 Data sequence patterns: In data compression, by sequentially predicting with high accuracy for each data point (e.g., equivalent to 1 bit of data) in the original data sequence the probability, based on past data points, that the data point will occur, data can be highly compressed. A data sequence pattern represents the probability that the predicted data point will occur.
*3 Comparison made under the same network configuration and other conditions, for autoregressive inference*4 assuming data compression, using approximately 12.6 million parameters in a conventional fast transformer-based approach*5 and approximately 2.8 million parameters in the inference model used with the newly developed technology.
*4 Autoregressive inference: Sequential prediction of the current data point by means of a statistical model with previous data points as inputs.
*5 Fast transformer-based approach: Among transformer-based approaches to data compression and data generation, a model designed for fast processing by adopting linear attention to reduce the computing volume.
For more information, use the enquiry form below to contact the Research & Development Group, Hitachi, Ltd. Please make sure to include the title of the article.
https://www8.hitachi.co.jp/inquiry/hitachi-ltd/hqrd/news/en/form.jsp